Products
OCHEM - our platform to create in silico ADME/Tox prediction models.
OCHEM makes it easy to create precise models for properties of chemicals.
- Webbased, completely integrated development system for Structure-Property- und Structure-Activity Relationships (QSPR, QSAR)
- Contains a large database of physical und ADME/T properties
- Requires only structural information
- Supports the identification of the mode of action
- Delivers background information for early stages of compound development
- Saves time and costs by allowing to eliminate redundant tests
- Allows distributed modeling in global teams
- Supports a wide variety of well-established machine learning and molecular descriptor programs.
- Associative Neural Networks, Fast Stagewise Multiple Linear Regression, K-Nearest Neighbors, Kernel Ridge Regression, SVM,
Partial Least Squares, Random Forests, Decision Trees
- E-state, ALogPS, MolPrint, GSFragment, Dragon, ISIDA, MOPAC, ADRIANA.Code, CDK, QNPR, ShapeSignatures, 'Inductive' descriptors,
MERA, MERSY, Vina Docking based descriptors, Chemaxon descriptors, Chiral Descriptors, ETM descriptors, Spectrophores
Learn more - or try it out yourself :
Wir offer a free version of OCHEM via the Federal Helmholtz Research Center in Munich
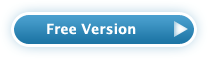
ePhysChem - fast and precise estimation of physicochemical and ADME/Tox properties.
Using ePhysChem, physical and ADME/Tox properties of chemicals can be estimated easily, quickly and precisely.
- ePhysChem contains AlogPS 3.01 for logP- and logS-prediction.
- ALOGPS 2.1 has been tested by various pharma companies and has repeatedly delivered excellent results (see VCCLAB).
- ALOGPS was recently benchmarked as the best off-the shelf package for the prediction of logP values on a set of in-house data from Pfizer & Nycomed - in comparison to 12 commercial and 6 public approaches (Mannhold et al, 2009).
- Application of the self-learning feature of our software can decrease the error further from 1.02 log units to 0.59 log units (Tetko et al, 2009).
(exemplaric on a test set of 95809 Pfizer-Molekülen)
- Moreover, our applicability domain approach allows to identify the 60% of the structures for which the predicition accuray reaches 0.33 log units.
- ALOGPS 3.01 was developed using the same approach, Associative Neural Networks, but on a 50% larger dataset of molecules for logP and almost 8 times larger dataset for water solubility.
- We are currently developing new modules for the prediction of DMSO solubility and pKa prediction, other important parameters in early drug research.
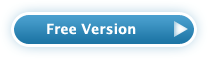